Is there a suitable plotting function allowing to avoid to compute and store the two potentially large arrays u
and v
, and rather provide just the function uv
(or equivalent)?
I'm not aware of a plotting function that just accepts uv
as input, but it seems moot. Whether we run uv
ourselves or the plotting function runs it, u
and v
will be computed and stored regardless.
Is there a better way to do this?
Yes, at least in terms of speed. Your code uses numpy.vectorize
, which is NOT vectorized since it's "essentially a loop" under the hood.
Faster options (see timing code at bottom):
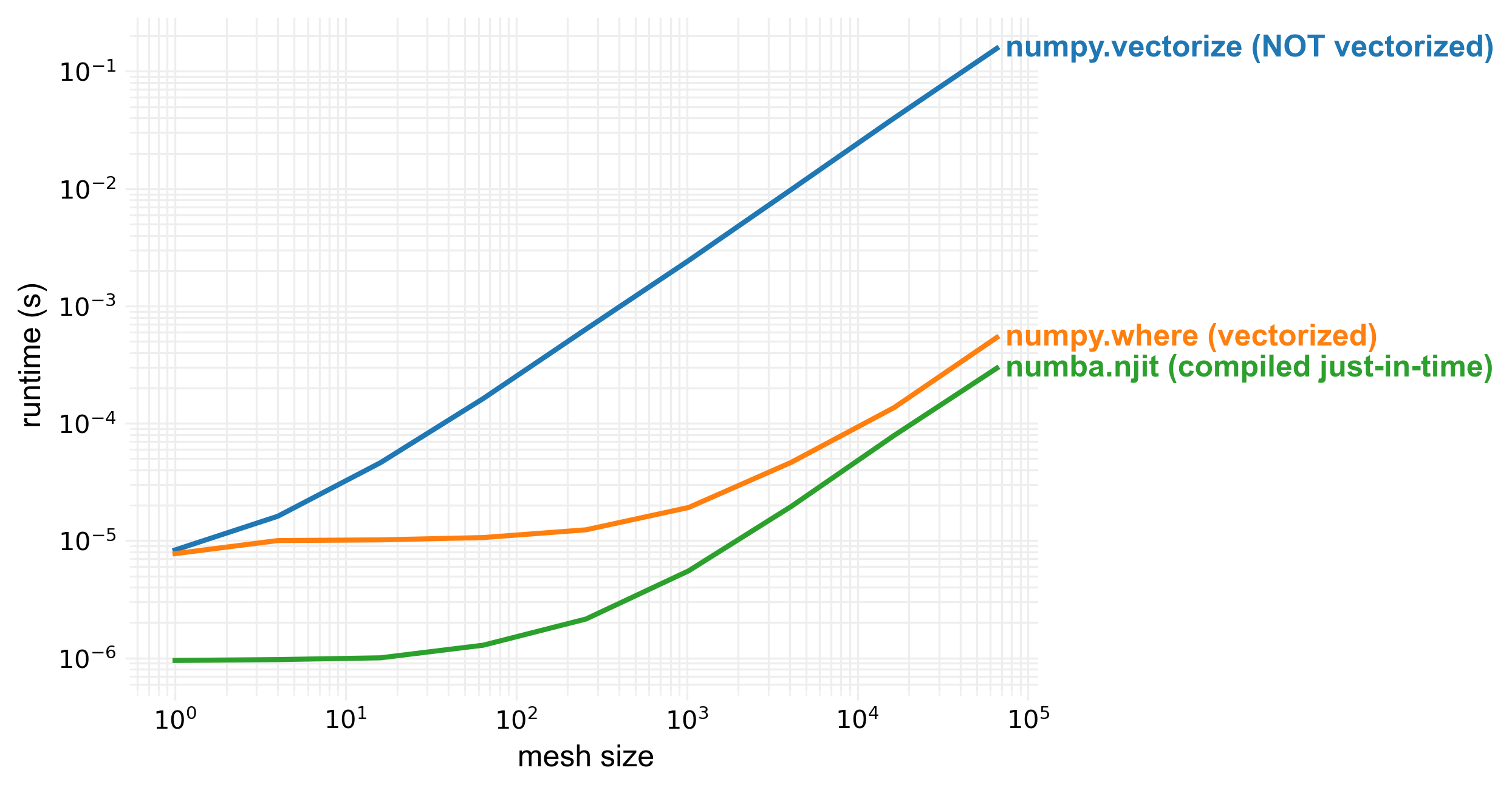
Vectorizing with numpy.where
(faster)
Given p
and q
from your lambda:
Y_sol = lambda r: np.array([1 - r/R_MIN, 1]) * np.exp(-r/R_MIN)
# ----------- - ---------------
# p 1 q
Your current code essentially iterates the following calculation since numpy.vectorize
is just a loop:
a, b = (p*q, 1*q) if R_MIN <= r <= R_MAX else (0, 0)
Instead it's faster to preprocess r
using numpy.where
and compute p
and q
all at once as arrays:
r_full = np.sqrt(x**2 + y**2)
r = np.where(
(r_full >= R_MIN) & (r_full <= R_MAX),
r_full, # if inside domain
np.nan, # if outside domain
)
p = 1 - r/R_MIN
q = np.exp(-r/R_MIN)
Looping with numba.njit
(fastest)
Looping is usually slower than vectorizing, but not with numba loops (i.e., decorated with numba.njit
) which get compiled on-the-fly into machine code.
Basic njit
version to compute u
and v
cell by cell:
@nb.njit
def solve_nb_njit(x: np.ndarray, y: np.ndarray) -> Tuple[np.ndarray, np.ndarray]:
u = np.empty((y.size, x.size))
v = np.empty((y.size, x.size))
for col in range(x.size):
for row in range(y.size):
r = np.sqrt(x[0, col]**2 + y[row, 0]**2)
if R_MIN <= r <= r_MAX:
p = 1 - r/R_MIN
q = np.exp(-r/R_MIN)
else:
p, q = 0, 0
u[row, col] = p*q*x[0, col] - q*y[row, 0]
v[row, col] = p*q*y[row, 0] + q*x[0, col]
return u, v
Revised code
In addition to the above changes:
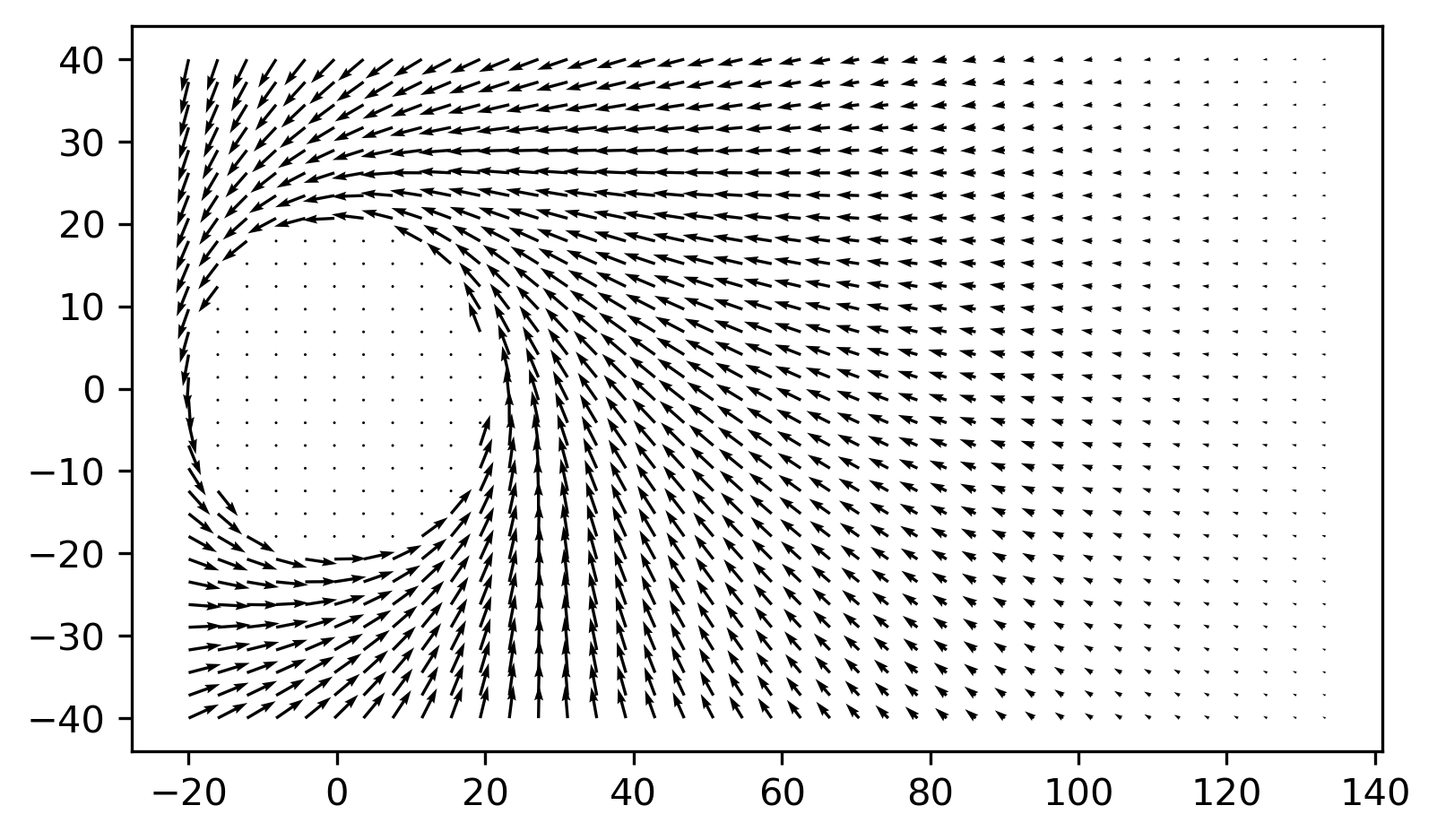
from typing import Tuple
import matplotlib.pyplot as plt
import numba as nb
import numpy as np
import pandas as pd
type Solution = Tuple[np.ndarray, np.ndarray]
def solve_np_where(x: np.ndarray, y: np.ndarray, r_min: int, r_max: int) -> Solution:
r_full = np.sqrt(x**2 + y**2)
# set domain all at once
r = np.where(
(r_full >= r_min) & (r_full <= r_max),
r_full, # if inside domain
np.nan, # if outside domain
)
# then the rest is just vector math
p = 1 - r/r_min
q = np.exp(-r/r_min)
u = q * (p*x - y)
v = q * (p*y + x)
return u, v
@nb.njit
def solve_nb_njit(x: np.ndarray, y: np.ndarray, r_min: int, r_max: int) -> Solution:
u = np.empty((y.size, x.size))
v = np.empty((y.size, x.size))
for col in range(x.size):
for row in range(y.size):
r = np.sqrt(x[0, col]**2 + y[row, 0]**2)
if r_min <= r <= r_max:
p = 1 - r/r_min
q = np.exp(-r/r_min)
else:
p, q = 0, 0
u[row, col] = p*q*x[0, col] - q*y[row, 0]
v[row, col] = p*q*y[row, 0] + q*x[0, col]
return u, v
def solve_np_vectorize(x: np.ndarray, y: np.ndarray, r_min: int, r_max: int) -> Solution:
def Y(r):
return np.array([1 - r/r_min, 1]) * np.exp(-r/r_min)
def uv(x, y):
r = np.sqrt(x**2 + y**2)
a, b = Y(r) if r_min <= r <= r_max else (0, 0)
return (a*x - b*y, a*y + b*x)
# NOT actually vectorized
u, v = np.vectorize(uv, otypes=[float, float])(x, y)
return u, v
def time() -> pd.DataFrame:
sizes = [2**k for k in np.arange(0, 18, 2)]
methods = {
'numpy.vectorize': solve_np_vectorize,
'numpy.where': solve_np_where,
'numba.njit': solve_nb_njit,
}
results = pd.DataFrame(index=sizes, columns=methods.keys())
for size in sizes:
print(size)
r_min = size * 0.02
r_max = size * 0.2
x, y = np.meshgrid(
np.linspace(-r_min, r_max*2/3, int(size**0.5)),
np.linspace(-2*r_min, 2*r_min, int(size**0.5)),
sparse=True,
)
for column, method in methods.items():
timing = %timeit -o -q method(x, y, r_min, r_max)
results.loc[size, column] = timing.average
results.plot(logx=True, logy=True)
plt.show()
return results