import timeit
import typing
import matplotlib.pyplot as plt
import numpy as np
import pandas as pd
import seaborn
def op(array: typing.Sequence[int]) -> tuple[int, int]:
""" returns the value of repeated number and missing number in the given array
using the standard formulaes of Sum of n Natural numbers and sum of squares of n Natural Numbers"""
missing_num = 0
repeated_num = 0
x = len(array)
sum_of_num = 0
sum_of_squares = 0
sum_of_num_actual = (x*(x+1))/2
sum_of_squares_actual = ((x)*(x+1)*(2*x+1) ) / 6
for num in array:
sum_of_num += num
sum_of_squares += num*num
missing_num = (((sum_of_squares_actual - sum_of_squares) /(sum_of_num_actual - sum_of_num))
+(sum_of_num_actual - sum_of_num))/2
repeated_num = (((sum_of_squares_actual - sum_of_squares) /(sum_of_num_actual - sum_of_num))
-(sum_of_num_actual - sum_of_num))/2
return repeated_num, missing_num
def sylvain_hjp_modified(array: typing.Sequence[int]) -> tuple[int, int]:
sum_of_num = sum(array)
sum_of_squares = sum(n*n for n in array)
x = len(array)
sum_of_num_expected = x*(x + 1)//2
sum_of_squares_expected = sum_of_num_expected*(2*x + 1)//3
# Assuming A is present twice and B is missing:
# B - A
b_minus_a = sum_of_num_expected - sum_of_num
# B^2 - A^2 = (B-A) * (B+A)
b2_minus_a2 = sum_of_squares_expected - sum_of_squares
# B + A
b_plus_a = b2_minus_a2//b_minus_a
a = (b_plus_a - b_minus_a)//2
b = (b_plus_a + b_minus_a)//2
return a, b
def numpy_arithpython_early_terminate(array: nptyping.ndarrayIterable[int]) -> tuple[int, int]:
sum_of_numincreasing = array.sumsorted(array)
sum_of_squaresmissing = array.dot(array)
None
xduplicated = lenNone
for x0, x1 in zip(arrayincreasing[:-1], increasing[1:]):
sum_of_num_expected if x0 == x1:
if missing is not None:
return x0, missing
duplicated = x*(xx0
elif x0 + 1)//2 == x1:
sum_of_squares_expected missing = sum_of_num_expected*(2*xx0 + 1)//3
# Assuming A is present twice and B if duplicated is missingnot None:
# B return duplicated, missing
def numpy_arith(array: np.ndarray) -> Atuple[int, int]:
b_minus_asum_of_num = sum_of_num_expectedarray.sum()
- sum_of_num sum_of_squares = array.dot(array)
#x B^2= -len(array)
A^2 sum_of_num_expected = x*(B-Ax + 1)//2
* sum_of_squares_expected = sum_of_num_expected*(B+A2*x + 1)//3
b2_minus_a2b_minus_a = sum_of_squares_expectedsum_of_num_expected - sum_of_squaressum_of_num
#b2_minus_a2 B= +sum_of_squares_expected A- sum_of_squares
b_plus_a = b2_minus_a2//b_minus_a
a = (b_plus_a - b_minus_a)//2
b = (b_plus_a + b_minus_a)//2
return a, b
def testnumpy_diff(array: np.ndarray) -> Nonetuple[int, int]:
forincreasing method= innp.sort(array)
diff = np.diff(increasing)
(i_dupe,), = (diff == 0).nonzero()
(i_miss,), = (diff == 2).nonzero()
return increasing[i_dupe], increasing[i_miss] + 1
METHODS = (
op, sylvain_hjp_modified, python_early_terminate, numpy_arith, numpy_diff,
)
def test() -> None:
for method in METHODS:
assert (1, 4) == method(np.array((5, 3, 2, 1, 1)))
assert (16, 7) == method(np.array((1, 2, 3, 4, 5, 16, 6, 8, 9, 10, 11, 12, 13, 14, 15, 16)))
# This test doesn't make sense: there is no missing value
# assert (1, 2) == method(np.array((1, 1)))
def benchmark() -> None:
times = []
for n in np.logspace(start=1, stop=5, num=50, dtype=int):
diffs = np.ones(n, dtype=int)
diffs[n//3] = 0
diffs[2*n//3] = 2
data = diffs.cumsum()
for method in METHODS:
def run():
return method(data)
t = timeit.timeit(stmt=run, number=1)
times.append({'method': method.__name__, 'n': n, 'time': t})
df = pd.DataFrame(times)
fig, ax = plt.subplots()
seaborn.lineplot(data=df, x='n', y='time', hue='method', ax=ax)
ax.set_xscale('log')
ax.set_yscale('log')
ax.set_title('Constant relative locations')
n = 1000
for loc in range(50, 1000, 50):
diffs = np.ones(n, dtype=int)
diffs[loc] = 0
diffs[loc + 2] = 2
data = diffs.cumsum()
for method in METHODS:
def run():
return method(data)
t = timeit.timeit(stmt=run, number=1)
times.append({'method': method.__name__, 'loc': loc/n, 'time': t})
df = pd.DataFrame(times)
fig, ax = plt.subplots()
seaborn.lineplot(data=df, x='loc', y='time', hue='method', ax=ax)
ax.set_title(f'Variable relative locations, n={n}')
plt.show()
if __name__ == '__main__':
test()
benchmark()
Later today I'll demonstrate a couple of other methods and benchmarks.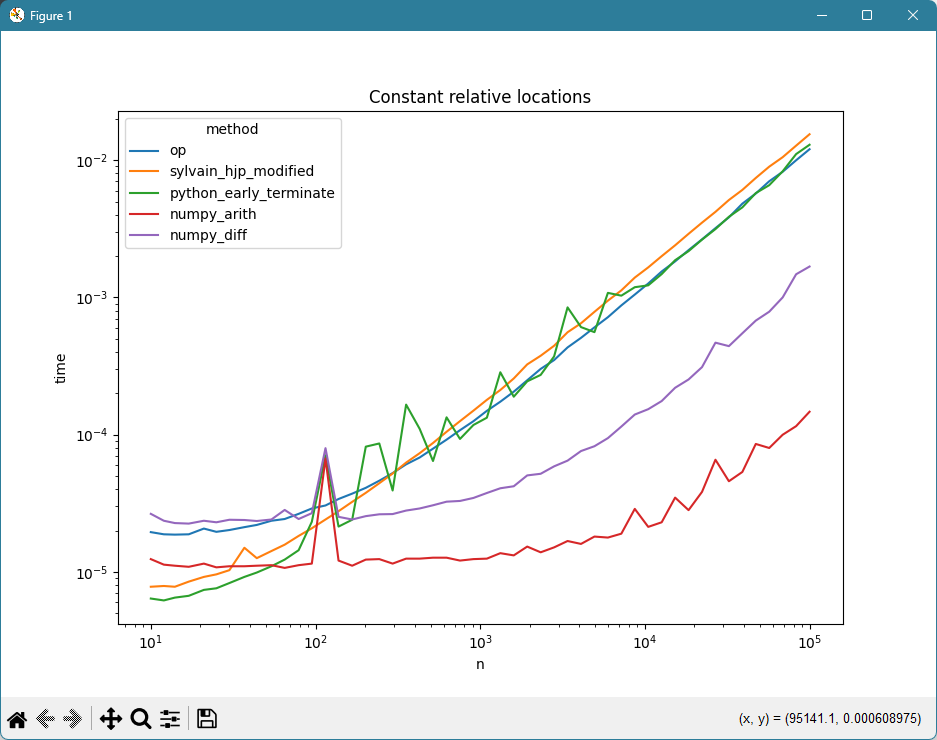
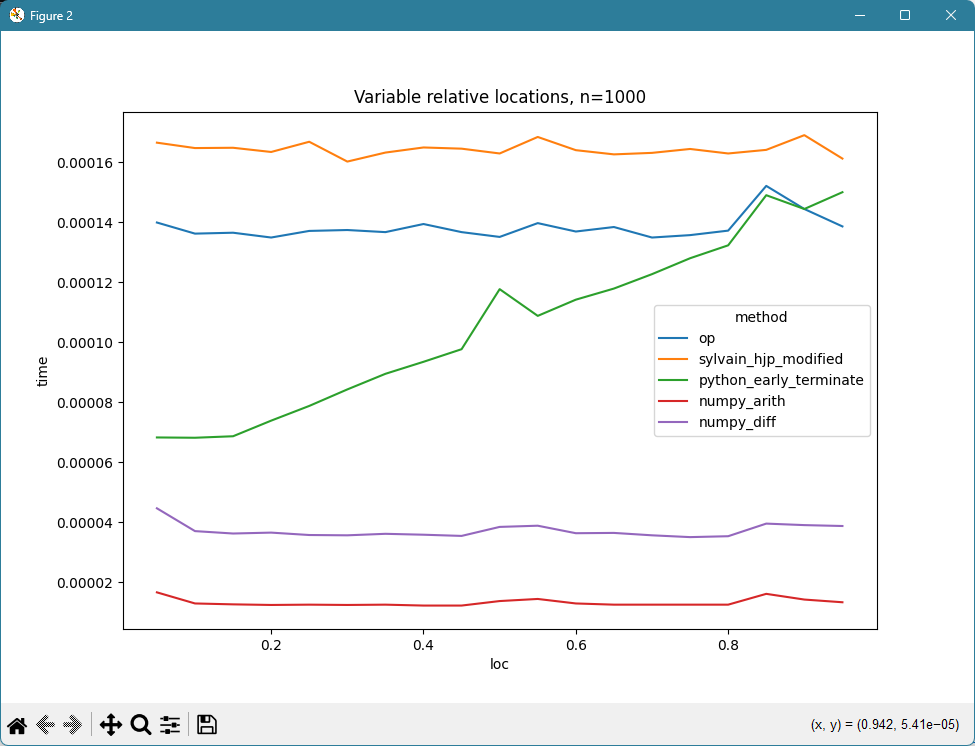